Big-O Cheat Sheet
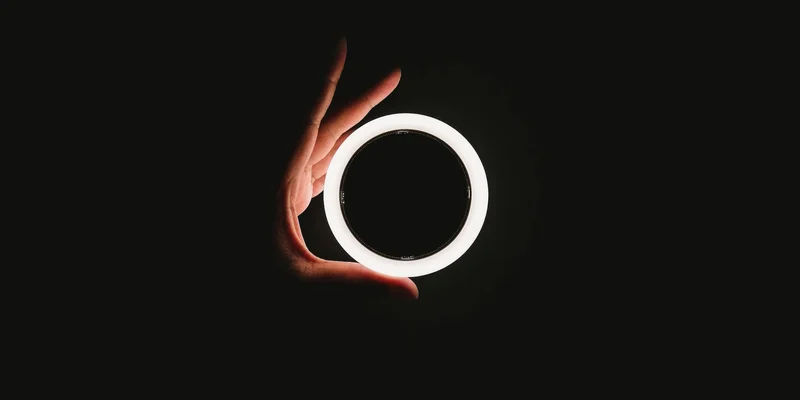
Definition
Big-O notation, represents an algorithm's worst-case complexity. It uses algebraic terms to describe the complexity of an algorithm, allowing you to measure its efficiency and performance. Below you can find a chart that illustrates Big-O complexity:
Simply put, O(1)
stands for constant time complexity, which is the most efficient, while O(n!)
stands for factorial time complexity, which is the least efficient. The n
in the complexity represents the size of the input, so O(n)
means that the algorithm's time complexity will grow linearly with the size of the input.
Apart from Big-O notation, there are other notations that are used to describe the complexity of an algorithm, such as Ω
(Omega) and Θ
(Theta). Ω
describes the best-case complexity of an algorithm, while Θ
describes the average-case complexity of an algorithm.
Common Data Structure operations
Different data structures have different time complexities for the same operations. For example, a linked list has O(1)
time complexity for insert
and delete
operations, while an array has O(n)
time complexity for the same operations. Below you can find average and worst time complexities for data structures used commonly in web development.
Average time complexity
Data Structure | Access | Search | Insertion | Deletion |
---|---|---|---|---|
Array | Θ(1) | Θ(n) | Θ(n) | Θ(n) |
Queue | Θ(n) | Θ(n) | Θ(1) | Θ(1) |
Stack | Θ(n) | Θ(n) | Θ(1) | Θ(1) |
Linked List | Θ(n) | Θ(n) | Θ(1) | Θ(1) |
Doubly Linked List | Θ(n) | Θ(n) | Θ(1) | Θ(1) |
Skip List | Θ(log n) | Θ(log n) | Θ(log n) | Θ(log n) |
Hash Table | N/A | Θ(1) | Θ(1) | Θ(1) |
Binary Search Tree | Θ(log n) | Θ(log n) | Θ(log n) | Θ(log n) |
Worst time complexity
Data Structure | Access | Search | Insertion | Deletion |
---|---|---|---|---|
Array | O(1) | O(n) | O(n) | O(n) |
Queue | O(n) | O(n) | O(1) | O(1) |
Stack | O(n) | O(n) | O(1) | O(1) |
Linked List | O(n) | O(n) | O(1) | O(1) |
Doubly Linked List | O(n) | O(n) | O(1) | O(1) |
Skip List | O(n) | O(n) | O(n) | O(n) |
Hash Table | N/A | O(n) | O(n) | O(n) |
Binary Search Tree | O(n) | O(n) | O(n) | O(n) |
Array sorting algorithms
Similar to data structures, different array sorting algorithms have different time complexities. Below you can find the best, average and worst time complexities for the most common array sorting algorithms.
Algorithm | Best | Average | Worst |
---|---|---|---|
Quick sort | Ω(n log n) | Θ(n log n) | O(n^2) |
Merge sort | Ω(n log n) | Θ(n log n) | O(n log n) |
Heap sort | Ω(n log n) | Θ(n log n) | O(n log n) |
Bubble sort | Ω(n) | Θ(n^2) | O(n^2) |
Insertion sort | Ω(n) | Θ(n^2) | O(n^2) |
Selection sort | Ω(n^2) | Θ(n^2) | O(n^2) |
Bucket sort | Ω(n+k) | Θ(n+k) | O(n^2) |